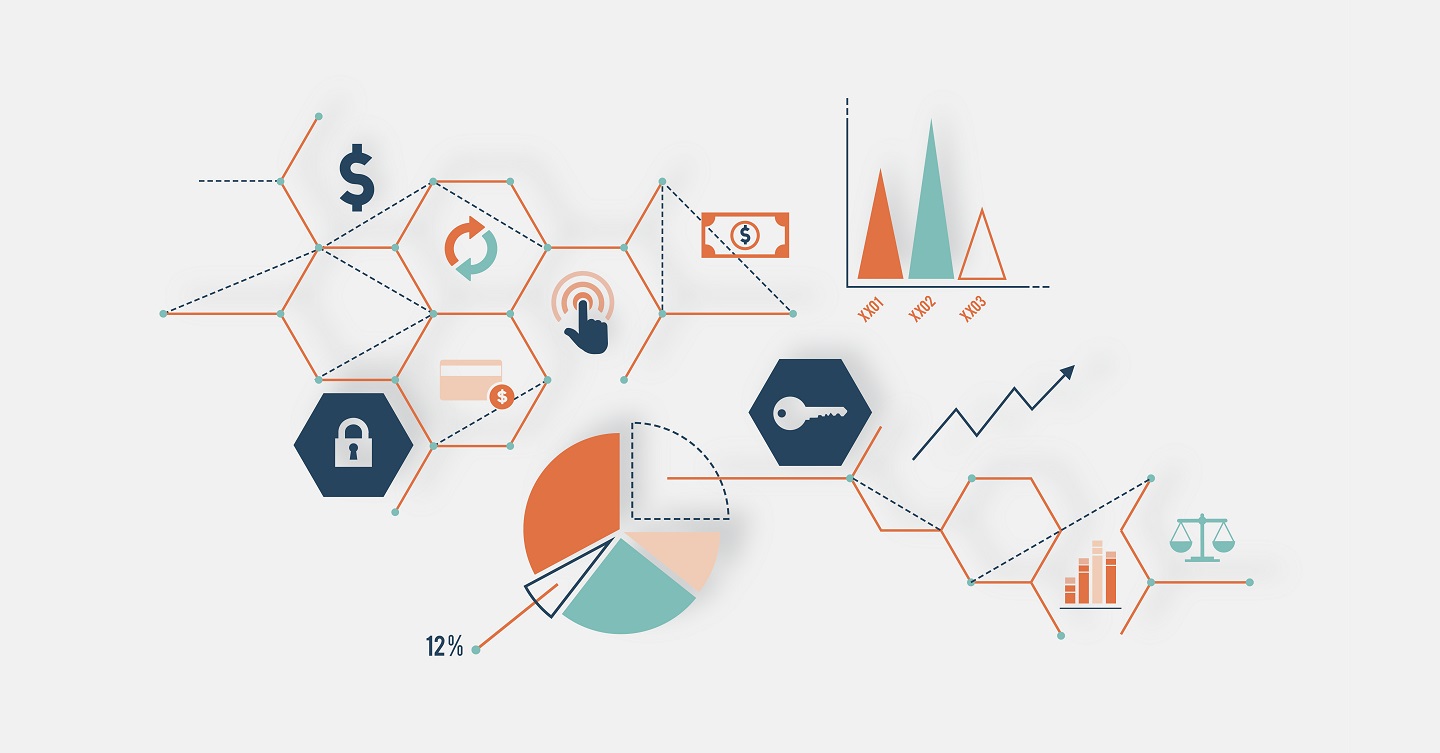
Strategic leaders are inundated with information that can possibly help them make decisions. This article is part 2 of a two-part series looking at how leaders can cull through and make sense of data and analysis and inform both their decisions and their bosses’ decisions. Part one looked at ideas such as who leaders should trust, different world views, and what is a construct. This part of the series addresses challenges with data and types and implications of errors in data analysis.
Leaders need to understand data–not just what it is, but how it is collected, processed, stored, and used.
Leaders often look to data to make decisions. Without doing due diligence to ensure the data is valid and reliable, important decisions can be skewed. Data needs to earn the right to be trusted, and leaders need to know how to build that trust in their data.
Mistakes We Make with Data
We are often told that data-informed decisions are better than decisions based solely on intuition. But data is not flawless and errors in data can lead to bad decisions. Leaders need to understand data–not just what it is, but how it is collected, processed, stored, and used. They then need to beware of common sources of error in data that can make it less valid and reliable and know how to ask the questions to build trust in their data.
Errors in data and analysis have a few causes. Some of these can be characterized as errors in measurement, and others can be characterized as errors in representation. Errors in measurement start with construct validity–simply put, how closely related is the data to the underlying construct of interest? For example, a study may measure a nation’s economic power using gross domestic product as its construct. Depending on the context, this may not be a valid construct as it misses other factors that may be important.
The next category of error in data is measurement error. These errors may occur when there is a problem with the measurement process. Anyone who has used a tape measure knows it matters where you clip the end of the tape. If you’ve burned a cake in an oven with a faulty thermometer, you know the impact of measurement error. To put it in terms used previously, biased scales or measurement processes can reduce the reliability and validity of your data.
Processing errors occur after the data is measured. If data is erroneously omitted or coded, analysis based on that data may be faulty. Effective data collection, cleaning, coding, and storage processes use quality control systems to minimize these errors. When looking at processing error, leaders should consider the incentives for the people collecting and entering data. Who are these people and what motivates them? Strategic decisions are often made on data that requires entry at the lowest level. What drives the teams entering the data? Think of the body count in Vietnam or Significant activities (SIGACTs) data in Iraq or Afghanistan. These data sources were used (perhaps inappropriately) to inform decisions at the highest levels of the U.S. government. Are battalions rewarded for complete and accurate data entry, or is data entry a low priority task? Are teams rewarded for skewing numbers one way or another? It is human to react to incentives. Good data collection methods have a system of quality control, where information is checked and corrected before being used for analysis. For example, in Afghanistan, a contracted quality control team working at the corps level checked SIGACTs data entered by battalions and brigades to reconcile, for example, transposition and missing digits in grid coordinates. Data must be trustworthy and accurate if analysis based on it is to be useful to senior leaders. Leaders should ask questions about data processing before making important conclusions based on the data.
Errors in representation have to do with how well the sample that is measured represents the population of interest. Making errors in representation can lead to unreliable and invalid data. The first representation error is sampling error. Unless you can measure the whole population of interest, sampling error is unavoidable. Without measuring the whole population, you won’t have the exact answer. Luckily for us, estimates based on representative samples allow for valid and reliable estimates without measuring an entire population. Statistics can inform you how large you need the sample to be to minimize sampling error to get a reliable estimate for the population. Simple online tools are readily available to help calculate the appropriate sample size.
A subset of sampling error is the problem of an N of 1. N of 1 refers to statistics where the sample size (N to statisticians) includes only one observation. The generalizability of statistics with only one observation is highly suspect; unless the population is completely uniform, a sample of one is not representative. So should you completely discount an individual experience? No, but you do need to recognize that when you read, “When I was a BDE CDR….” that the data that follows is from a limited source and may or may not be a representative sample. We tend to overvalue our personal experiences, and so this is an issue we must be conscious of as consumers of information.
The next error in representation is coverage error. Coverage error occurs when the list (called frame by statisticians) that we chose our sample from is not an accurate representation of the population that we are interested in. Data often does not cover the entire population. Potential holes in data coverage can limit the data’s usefulness for decisions. For example, the International Security Assistance Force (ISAF) coalition in Afghanistan contracted for population surveys to understand the Afghan people’s perceptions about security and governance. But polling data in Afghanistan often missed the most violent districts where surveys could not be administered safely. In a few other districts, samples did not include women due to cultural constraints. Leaders need to understand limitations in data coverage when using the data to inform decisions.
Non-response error is specific to survey data and occurs when not everyone in the sample participates in the survey. If the non-respondents are meaningfully different from the respondents, then the measurement will be biased. If the people who responded to the survey are different than those that did not, the measurement will not be an accurate estimate of the population.
Finally, there is the adjustment error, and it often occurs when the researcher is analyzing the data. This occurs when the researcher adjusts the measure to account for other sources of representation error. These adjustments are frequently done in population surveys, and to do them effectively requires skill and judgment. For instance, if males were underrepresented in the response rate compared to their existence in the population, researchers might put additional weight to the answers of the males that did respond. Inaccurate adjustments can add additional error into the data.
Population surveys informed strategic decisions during missions in Iraq and Afghanistan. But how do you know that the data/information that you are receiving from a survey is valid and reliable?
Cautions About Survey Data
Survey data is an important subset of data often used to inform senior leaders. You have likely filled out countless surveys from command or workplace climate to customer service over the course of your professional careers. Population surveys informed strategic decisions during missions in Iraq and Afghanistan. But how do you know that the data/information that you are receiving from a survey is valid and reliable? Errors can occur in the most stringent, peer-reviewed, academic research as well as the casual survey taken by a local news channel.
Understanding survey design and sources of error can help you frame questions to understand how the researchers are minimizing these errors. By asking a few simple questions, you can evaluate survey validity. Identifying surveys that were built to support a specific point of view (for example, one that asks leading questions making it clear that there is a correct answer) or ones that were conducted in a manner to measure the construct with the least possible errors is a valuable skill for leaders analyzing data.
Type I/Type II Errors.
Statisticians talk about two important types of errors. Type I errors are false positives—you identify a condition when it does not exist. Type II errors are false negatives—you fail to identify a condition when it really exists. Both types of error can result in bad outcomes.
So what? If you are not a statistician, why do you care? An example: Consider your spam filter on your email account. The baseline assumption, called the null hypothesis by statisticians, is that any email that you may receive is an email that you want. The spam filter’s job is to identify emails that are not desired. A Type I error would be when your spam filter identifies “good” email as spam and sends it to the spam folder. However, if the same filter lets a spam email through to your inbox, then you just experienced a Type II error.
How you conduct your statistical test can make one of these errors more likely. Tune your test to minimize false positives and you may miss true positives. Set your tolerance the other way and you will get more false positives. Leaders should understand this concept so they can converse and provide guidance to analytic teamsas even the most meticulously conducted research is subject to Type I and Type II errors. There is no expectation that terms like “reject the null” or “type I error” will permanently enter your lexicon, but we do hope that you realize that researchers make choices and decide to accept or reject their analysis based on the current conventions and methods accepted in their respective fields.
Challenges with data and the errors possible in data analysis are critical for leaders to understand when they are attempting to use data to inform decisions. We know that this two-part series will not make data scientists out you, but like it or not, you are inundated with data. People will attempt to use data to influence your thinking as well as inform your decisions. You will probably do the same for your boss. After all, as Andrew McAfee stated, “The world is one big data problem.”
COL Jeffrey E. Baker is an Army Officer and instructor in the Department of Command, Leadership, and Management at the U.S. Army War College.
Bob Bradford is a retired U.S. Army colonel and the Professor of Defense and Joint Processes in the U.S. Army War College’s Department of Command, Leadership, and Management.
The views expressed in this article are those of the authors and do not necessarily reflect those of the U.S. Army War College, the U.S. Army, or the Department of Defense.
Photo Credit: Infographic vector created by rawpixel.com – www.freepik.com