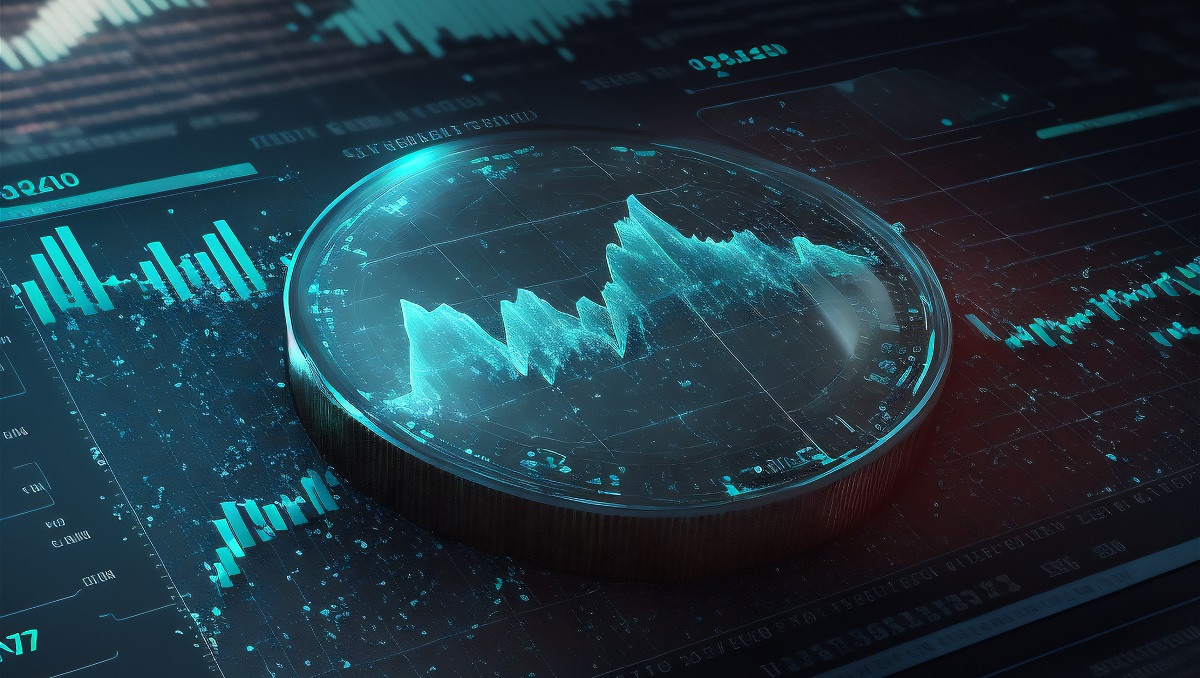
A cynic might declare that data-centric operations are nothing more than an old concept made new.
In a May 2021 memorandum to senior leaders, Deputy Defense Secretary Kathleen Hicks declared that data was a strategic asset, and she issued a clarion call to transform the Department of Defense into a “data-centric organization.” Her memorandum directed the DoD to seek ways to make data more accessible and easier to integrate. To this end, the Army subsequently issued its Data Plan as an outline for moving forward, and this plan describes no less than ten strategic objectives to become “data-centric.” The intent is to use data to make decisions that will “outpace an adversary” and win future conflicts.
A cynic might declare that data-centric operations are nothing more than an old concept made new. As early as 1993, the Army embraced the post-Cold War Force XXI transformation model, which acknowledged that information technology would revolutionize warfare. The revolution was ostensibly the introduction of networked information technology systems that enabled commanders to make decisions faster, dominate the battlespace, and win throughout the full spectrum of operations. TRADOC Pam 525-5 Force XXI Operations was even ahead of its time in 1994 when it identified in one paragraph a trend toward “brilliant systems” that used AI to improve operations, intelligence, and logistics capabilities. The pamphlet even predicted that the health of individual soldiers might be monitored. However, the latest call for transformation is different in that it recognizes that information systems (e.g., Maneuver Control System, All Source Analyst System, Command Post of the Future, etc.) are important but not as important as the data itself.
To fully embrace data-centric operations, however, one must grapple with complex data science terms such as biomes, labeling, big data, clustering, decision trees, neural networks, and the list goes on seemingly ad infinitum. Thus, the complexity of data terms can become overwhelming and make data neophytes of the most seasoned military officers. Indeed, discomfort with data analytics is not only confined to military professionals. In his book Be Data Literate: The Data Literacy Skills Everyone Needs to Succeed, James Morrow cites a 2019 study indicating that a mere 32 percent of civilian business executives were “able to create measurable value from data” and only “27 percent said their data and analytics projects produce actionable insights.” Frustration over data integration, according to Morrow, often leads civilian counterparts to give up data-driven operations in favor of doing things the “old way.” However, this is not an option for military professionals. China is investing heavily in data to create AI-enabled autonomous vehicles, ISR platforms, and predictive logistics capabilities that will enable it to leverage swarm technology, sense U.S. military maneuvers, and sustain operations over long distances more effectively.
Given these emerging threats, commanders and their staff officers are seeking to understand and implement data into operational platforms. Thus, a straightforward and natural question often emerges in the mind of these military professionals: “I need to become data literate, but where do I start?” The good news is that becoming a data scientist is not a requirement, but having a mental model to navigate through the concepts is useful. Daniel Jones discusses in his book Data Analytics: A Comprehensive Guide to Learn and Understand Data Analytics and Its Functions the importance of skillset, mindset, dataset, and toolset in data analytics. Thus, I propose set convergence as an approach that might help data neophytes begin their journey. Convergence is a term borrowed from design thinking in which various ideas are filtered to generate new perspectives and features prominently Joint Operational Design and Army Design Methodology. Set convergence is simply the suggestion that data-centric operations are the convergence of skillset, mindset, dataset, and toolset. If a neophyte approaches data-centric operations with this model, he or she will have a good foundation for sorting through the complexities surrounding data thinking. The model itself does not hold all the answers to the data puzzle, but it helps to begin asking the right questions.
Skillset is arguably the easiest part of the model to obtain. The Army is full of trained and educated specialists who routinely generate data. Logisticians order supplies. Doctors treat the wounded. Intelligence officers analyze information and so on. However, a challenge often arises when practitioners do not understand that they are also Data Generators and the relevance their data has in operational decision-making. Thus, you are not just an Infantryman; you are an Infantryman who is a Data Generator. This might sound like a trivial distinction, but it is an important adjustment in self-perception that is necessary for data-centric operations. It also requires the practitioner to understand that her data is important to the overall operation. Too often skilled practitioners mistakenly perceive their data as specialized and unrelated to the larger operational approach, but all data sets are worthy of examination. Once a practitioner fully understands the significance of being a Data Generator, he or she can develop a mindset that looks at data differently.
Dataset identification might be the most difficult challenge, but data neophytes often need only to explain and provide information to professionals who assist units in the development of data capabilities.
Mindset is the ability to identify and read data that might describe, diagnose, predict, and/or prescribe. Is the data categorical, quantitative, temporal, or spatial? Is your data structured in an Army system of record, or is it unstructured data residing on an obscure and inaccessible file server? Is it semi-structured within a spreadsheet or database? Once data neophytes ask these types of questions, they are demonstrating an ability to “read data.” The Center for Data Analysis and Statistics at the United States Military Academy is leading the way in developing introductory training in data literacy, and the Army should continue to invest in such programs in tactical, operational, and strategic level formations. Obtaining a basic level of data literacy helps data neophytes identify datasets.
Dataset identification might be the most difficult challenge, but data neophytes often need only to explain and provide information to professionals who assist units in the development of data capabilities. In some cases, these professionals are vendor representatives who are fielding new equipment, but the Army is also investing in the hiring of civilian employees who are data scientists and help organizations with data requirements. For example, the XVIII Airborne Corps has two government employees who serve as Chief Data Officer (CDO) and Chief Technology Officer (CTO). To effectively communicate with these professionals, one should be able to converse around some basic questions:
- Is the data transparent? Where is the data coming from, and is it trustworthy? This might seem an easy question to answer but imagine working with a partner force in a deployed environment. For a plethora of reasons ranging from time available to language barriers, the data you are ingesting might be flawed and lead to faulty analysis.
- Is the data precise? In other words, does the data answer a specific operational question? The only reason to consider data is if it helps the commander make decisions. It is important to understand that the data you generate might not prima facie have direct operational implications; however, your data might intersect with other data in a way that leads to precise deductions. Again, the mindset that all data is important and worthy of examination is important.
- Is the dataset large enough? Distinguishing between reoccurring patterns and anomalous events is extremely challenging, especially given that anomalous cases occasionally have outsized effects. Data sets should ultimately be representative of trends in the operational environment and not overly influenced by anomalous cases.
- Is the data timely? Live data should be the gold standard for data-centric operations, and the ability to effectively integrate live data into operational decisions will determine the outcome of future conflicts. Because all data is important and relevant beyond the scope of a particular warfighting function, data should, if possible, reside in cloud-based environment. If it is cloistered on computer hard drives or buried deep in a file server, it becomes useless.
These are rudimentary questions to help the data neophyte begin conversations with data scientists; and as data literacy increases, the sophistication of interactions will also increase. Pairing up practitioners, software engineers, and data scientists in exercises such as the XVIII Airborne Corps’ Scarlett Dragon has enabled the development of cutting edge data-centric capabilities to significantly improve the speed of targeting capabilities.
Nearly every warfighting function has a toolset for assisting in task execution. In fact, there are hundreds of systems and applications in the Army inventory. These tools may sense (input layer), apply algorithms (hidden layer), or produce deductions (output layer). Sometimes toolsets do all three at once. To conduct data-centric operations effectively, however, systems need to be open standard and interoperable. Proprietary systems that do not interact with other systems are sub-optimal. To avoid these conflicts, contracting processes should mandate interoperability in statements of work. Whenever it is possible, organizations should seek to purchase the data used on its systems because the data is more important than the system. This is a challenge given that many dataset owners are often reluctant to work with DoD entities. Still, when it is possible, DoD organizations should prioritize dataset ownership over dataset consumption. Set convergence is “a way” in which to start thinking in new ways about data-centric operations. Using tools developed Exercise Scarlett Dragon, the XVIII Airborne Corps merged skillset, mindset, dataset, and toolset in the summer of 2022 to successfully advise and assist allies and partners. Lessons learned from this deployment are being disseminated throughout the force to prepare for future conflicts. Given China’s embrace of data-enabled operations, it is imperative that leaders receive these lessons, and set convergence is a model that might help convert data neophytes into data-centric operators.
Jerry Landrum is a 2000 graduate of the University of North Georgia and a colonel in the U.S. Army. His last assignment was with the XVIII Airborne Corps at Fort Liberty where he was introduced to data-centric operations and observed the practical implementation of JADC2. Colonel Landrum attended Kansas State University where he earned a PhD in Security Studies. He is currently serving as a Faculty Instructor at the United States Army War College. COL Landrum was a member of the Carlisle Scholars Program and a graduate of the AY22 Resident Course at the U.S. Army War College.
The views expressed in this article are those of the author and do not necessarily reflect those of the U.S. Army War College, the U.S. Army, or the Department of Defense.
Photo Credit: Image By svstudioart