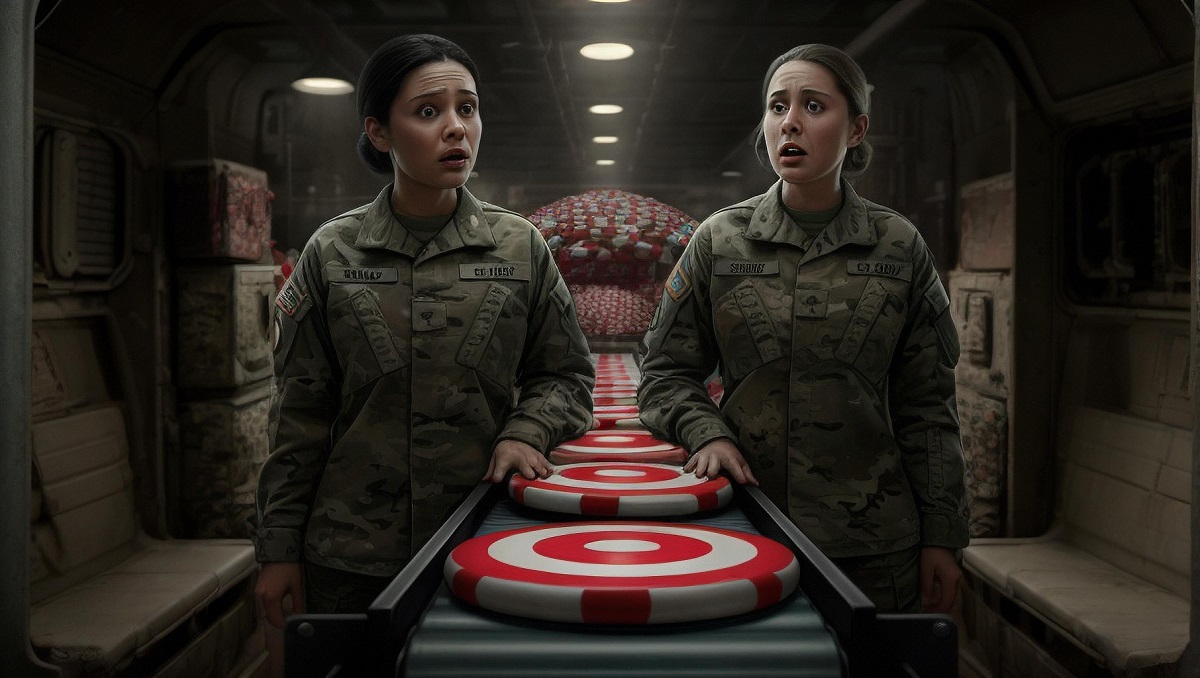
Editor’s Note: This is the second installment of a three-part series delving into the role of artificial intelligence (AI) within the United States’ comprehensive national defense and security strategy. The authors will assess the advantages and limitations of AI as it is employed to enhance, integrate, and potentially supplant human decision-making processes. You can find the first article here.
Who do you think you are / Ha ha ha, bless your soul / You really think you’re in control
— “Crazy,” Gnarls Barkley
It is popular to argue that the future of artificial intelligence (AI) is “humans with AI” (HWAI), which Harvard Business School Professor Karim Lakhani neatly summarized: “What I say to managers, leaders, and workers is: AI is not going to replace humans, but humans with AI are going to replace humans without AI.” The continued centrality of human decision-makers is a nice, comforting idea, and it will probably work in certain kinds of future operational environments. But HWAI will almost certainly not work in the emerging environment of high-intensity warfare.
In an episode of I Love Lucy, Lucy and her friend Ethel find themselves working in a chocolate factory. Tasked with wrapping individual chocolates moving on a conveyor belt and ensuring no unwrapped treats get through, Lucy and Ethel quickly fall behind as the belt starts to accelerate. The women start to panic, desperately trying to wrap the chocolates before they disappear down the line. Soon, they resort to hilarious tactics, as Ethel starts popping chocolates in her mouth to hide them from the stern supervisor, and Lucy begins stuffing them in her pockets, blouse, and hat. The chaos escalates, with candy flying everywhere as they are overwhelmed. When the supervisor returns to check on Lucy and Ethel’s work, she’s pleased to discover that no unwrapped chocolates made it through. “Speed it up a little,” she yells to the person controlling the conveyor.
Faced with an impossible situation, Lucy’s progression from frustration to desperation mirrors the psychological journey of many decision-makers in high-pressure environments, who may also resort to increasingly drastic measures to cope with cognitive dissonance: psychological discomfort created by the gap between their beliefs and their actions (more on this below). Lucy’s passage from constructive, honest coping with this dissonance to destructive, dishonest coping occurs in seconds and serves a great comedic purpose. In the real world, this change can be gradual or rapid, depending on the situation and the decision-maker. More to the point, we believe that this dissonance-driven journey has lessons for the design of future AI-enabled military decision systems.
The U.S. military’s HWAI paradigm for the role of human decision-making in AI-enabled war rests on unrealistic assumptions about how humans will think and interact with advanced AI technologies. Whatever modest advantages humans may have in decision-making will likely be negated by the reality of operating in machine-speed decision systems. In future wars, as human decision-makers struggle to relieve system bottlenecks by bundling decisions, deferring excessively to AI recommendations, altering system parameters without permission, or enacting other unanticipated behaviors (including outright deception), they will undermine the systems’ functions. Making timely human-controlled decisions in such systems will require behavioral improvisations that at best maintain an illusion of human control but accept de facto control by machines, and at worst conceal failures as decision-makers frantically stuff chocolates into their hats. Before we elaborate on this argument, we should first describe the “humans with AI” paradigm of military decision-making. What are current U.S. expectations for how humans will work with AI in war?
Human Augmentation: The HWAI Approach
In the HWAI framework, AI will assist but not replace key human decision-makers in war. As scholar Anthony King writes, “When massive data sets are processed effectively by AI, this will allow military commanders to perceive the battlespace to a hitherto unachievable depth, speed and resolution.” AI enhances and augments human capabilities in war, but should not be empowered to make high stakes decisions such as the use of lethal force. In November 2023 remarks on the “State of AI in DoD,” Deputy Secretary of Defense Kathleen Hicks explained, “AI-enabled systems can help accelerate the speed of commanders’ decisions, and improve the quality and accuracy of those decisions—which can be decisive in deterring a fight, and in winning a fight.” Addressing concerns about the role of AI in war, Secretary Hicks reiterated the United States’ position on lethal autonomous weapons: “As I’ve said before, our policy for autonomy in weapon systems is clear and well-established. There is always a human responsible for the use of force. Full stop.” In this view, military AI may be used as a decision aid in high-stakes decisions, but it will not make final choices on using force or risking the lives of personnel. These decisions will remain under human control, keeping a “human in the loop” for lethal and other high-stakes decisions, per current DoD policy.
HWAI has a simple, four-part logic of:
- Humans have valuable decision-making capabilities that machines cannot and will not replicate.
- Machines have valuable decision-making capabilities that humans cannot and will not replicate.
- The combined set of capabilities of humans and machines working together exceeds the set of capabilities of either humans or machines working separately.
- Therefore, humans and machines working together will outperform humans or machines working separately.
But what if the fourth proposition is wrong? What if the integration of human decision-makers into machine-speed decision systems compromises the systems’ functions? In a prior essay, we expressed skepticism about the supposed inherent superiority of human decision-making. But even if we accept that premise, decision quality is not solely dictated by the quality of the decision engine (e.g., the human brain versus a machine intelligence). The context of decision-making also matters, and advanced, AI-enabled kill chains will produce decision contexts uniquely ill-suited to direct human control. Such systems that rely on humans will exhibit a three-step tendency to deteriorating performance.
Step 1: High Demand for Fast, Complex Decisions
Modern battlefields will generate vast amounts of complex information, especially in high-intensity conflict. AI-enabled, high-intensity conflict will feature an accelerating tempo and increasing complexity of operations. This will result from three key developments, all already well underway: the proliferation of sensors and data, the mass deployment of autonomous systems, and the complexity of networked operations.
As a result of these three factors, the cycling time of the kill chain will accelerate even as the kill chain itself grows in complexity. The decision requirements of these systems will be faster and more complex. Amidst all this, human decision-makers will also have less control over the clock.
Human delays in HWAI systems will tend to severely degrade overall system performance, especially when the system relies on masses of unmanned sensors and shooters. In such environments, even minor human delays can disrupt the synchronization between these autonomous elements, leading to missed opportunities or erroneous actions. Those delays will then compound as each subsequent decision becomes increasingly outdated, causing cascading failures in the system’s operational effectiveness. This not only risks operational success, but also undermines the advantages of speed, efficiency, and scalability provided by the integration of AI and autonomous technologies.
To relieve the bottlenecks, decision makers will have to do something different.
Step 2: Decision Bottlenecks Form
The constant influx of information, demand for decisions, high speed of operations, and heightened complexity of modern warfare will place immense pressure on human decision-makers who find themselves facing a relentless conveyor of decisions. Without being able to slow the “decision clock,” a bottleneck will form. Under these conditions, decision-makers risk cognitive overload, fatigue, and impaired judgment, resulting in bad decisions, delayed decisions (which may be worse than a bad decision), or no decisions at all. The desire to get away from the conveyor will be strong.
Machines and automated processes can handle information and tasks at speeds limited only by hardware and energy. Given humans’ natural limitations of speed and processing ability, we simply can’t make decisions at the same speeds as a machine. Israel has reportedly used an AI-enabled system called Lavender for selecting bombing targets in Gaza. One intelligence officer who used the system described how it affected the volume of decisions: “Because of the system, the targets never end. You have another 36,000 waiting.”
To relieve the bottlenecks, decision makers will have to do something different.
Step 3: Clearing the Bottleneck through Improvisation
In impossible decision-making environments such as the HWAI system we just described—where decisions need to be made both quickly and under conditions of high complexity, without sufficient time for proper analysis—cognitive strain (sometimes called “processing disfluency”) will accumulate and become acute cognitive dissonance—a psychological discomfort arising when we hold two or more contradictory beliefs, ideas, or values, or when our actions are inconsistent with our beliefs. When Lucy struggled to keep pace with the increasingly rapid conveyor belt in the chocolate factory, she experienced dissonance between her expectations and her actual performance wrapping chocolates.
Humans do not like cognitive dissonance. In cases in which dissonance involves a gap between our beliefs and our actions, we can close the gap by changing our beliefs or changing our actions. Lucy first tried to reduce the dissonance by setting chocolates aside, but the pile of unwrapped chocolates just grew. Finally, in desperation Lucy resorted to outright deception.
Similarly, humans at key decision points in HWAI kill chains in high-intensity conflicts will try to relieve the pressure of their situation. They will have three (not mutually exclusive) options: (1) seek help, (2) give up, or (3) improvise new behaviors. Seeking help may be effective in some situations, but in the most acute circumstances, the compounding effects of the volume, pace, and complexity of decisions will likely overwhelm decision-makers throughout the system. It’s hard to find a helping hand when everyone needs help. Giving up is deeply counter-cultural in the military. What about improvising new behaviors?
Humans have a great capacity for behavioral surprises. The question is how these improvisations will affect the performance of the system. Behavioral improvisations will likely undermine HWAI systems by introducing unanticipated decision criteria as decision-makers deviate from standard procedures. As decision-makers rely on AI to do things it was not designed to do, they increase the risk of failure. But what do these improvisations look like in practice? We cannot foresee all possible types, so we’ll focus here on three examples: aggregation or bundling of decisions, excessive deference to the AI, and modifying system parameters without permission. All three are problematic.
Bundling AI Recommendations into Human Decisions
Relieving the bottleneck by aggregating or bundling lower-level AI recommendations into higher-level human decisions inevitably reduces human decision-makers’ awareness of the content of their decisions. In effect, bundling cedes control of key lower-level decisions to an AI, involving a human only at a higher level of approval. The decision system becomes a de facto supervisory “human on the loop” approach, even though we may still claim to have a “human in the loop.” By insisting on remaining central, we may lie to ourselves about the fidelity of our understanding.
Excessive Deference to the AI
Relieving the bottleneck through excessive deference to AI recommendations is even more problematic. It will be very tempting for human decision-makers simply to accept AI recommendations without applying appropriate scrutiny by following established procedures. Another user of Israel’s Lavender AI system recounted, “I would invest 20 seconds for each target at this stage, and do dozens of them every day. I had zero added value as a human, apart from being a stamp of approval. It saved a lot of time.” As with bundling choices, excessive deference to AI blurs an important distinction between human and machine roles in the system, and this reduction of role clarity is likely to degrade the quality of decisions. Again, when decision-makers defer to AI beyond its intended role, the AI effectively becomes the decision-maker for decisions it was merely designed to inform.
Modifying System Parameters
A third form of improvisation is directly modifying (without permission) the parameters of the AI system itself. In an AI-enabled military command and control system, these parameters may include aspects of the system’s user interface, as well as the rules and criteria for presenting decisions to humans in the loop. In high-intensity operations with a mounting backlog, decision-makers may try to modify these parameters to relieve pressure. For example:
- Decision priority parameters determine the urgency of each decision, from critical to routine. A decision-maker may adjust priority settings to move decisions to lower levels.
- Time sensitivity parameters set the time for decision-maker to respond to different decisions. A decision-maker may extend response times to make certain types of decisions.
- Information scope parameters control the amount and type of data presented to decision-makers, ranging from detailed to summary views. A decision-maker may lower the scope of information, reducing data complexity to speed up decision-making.
- Automation level parameters specify which decisions are handled by the AI autonomously versus requiring human input. A decision-maker may lower automation thresholds, allowing the AI to handle more decisions automatically.
These modifications may solve decision-makers’ immediate problems, but they are likely to create bigger future problems. Lowering the priority of decisions or extending response times can disrupt the system’s ability to sequence and coordinate actions in the broader system, affecting other critical actions. Reducing the complexity of information presented to decision-makers may hide key factors needed to understand the full context of a situation, increasing the likelihood of poor decisions. Lowering decision thresholds for automation might cause the AI to misinterpret situations or handle decisions it is not fully equipped to manage, leading to unsafe outcomes.
This tendency to solve a near-term problem by creating a bigger, nastier long-term problem is the main risk arising from all three improvisations just named—and from improvisation in general. Unanticipated behaviors will tend to change and corrupt the system in unexpected ways. Charles Perrow’s concept of “normal accidents” captures how strongly interdependent (which Perrow calls “tightly-coupled”), complex systems are prone to failure. Small, seemingly manageable errors can rapidly become much larger problems. Improvisations such as bundling, excessive deference, and messing with system parameters aggravate the risk of these accidents. When decision-makers do these things, they introduce risk beyond their specific portfolio of decisions due to the tight coupling and dense interactions of the system. Even small deviations can interact unpredictably with the system’s human and AI decision processes, leading to unforeseen outcomes and potentially catastrophic failures. The integrity of the entire decision-making process is compromised when human operators misrepresent their actions.
Furthermore, such modifications often become routine because they seem to solve an urgent problem for decision-makers. Yet over time these changes tend to create problems that may compound and grow unseen, especially in AI systems designed to learn. As these hidden issues accumulate, they may eventually explode into much larger crises that prove devastating.
Conclusion
The development and use of empowered military AI systems capable of lethal decision-making without human intervention is probably inevitable. Appealing as it is for humans to remain in control of key decisions, humans will create bottlenecks in advanced AI-enabled kill chains where rapid analysis and coordination are essential for operational success. Relying on human approval throughout the engagement process risks delayed reactions and outdated AI recommendations within fast-evolving battlefields. Is this increase in risk to the mission offset by human control of the decisions, especially when the solutions to the bottleneck problem degrade the quality of those decisions?
Perversely, an AI designed to make decisions that are sensitive to risks to humans may produce more compassionate choices than a human making the same choices using an AI that is only designed for decision support. Recent research on how humans interpret AI recommendations also suggests that HWAI systems may have strange effects on human decision-making. Research by Shane Schweitzer and others presented at a recent symposium found that decision-makers judged job applicants as less human (i.e., they dehumanized the applicants) when they were told that the applicant was simply selected by an algorithm, compared to when they were told that the applicant was selected by a human analyst. For example, applicants in the algorithm-selected group were judged to be less capable of complex thoughts and emotions, and more robotic.
The history of military innovation suggests that we may have to learn some painful lessons in actual conflict before we understand the answer, but we believe that the accelerated tempo of warfare driven by AI will demand a new approach to managing military power, where speed and complexity must redefine the boundaries of human control.
We take no pleasure in arguing that the U.S. military’s commitment to the humans-with-AI approach is based on questionable assumptions about human integration with advanced AI systems in war. We would prefer to live in a world in which such things were the exclusive concern of science fiction. But they are not, and it is essential that we confront the world as it is, and as it is becoming.
Andrew Hill is Professor of Strategic Management in the Department of Command, Leadership, and Management (DCLM) at the U.S. Army War College. Prior to rejoining the War College in 2023, Dr. Hill was the inaugural director of Lehigh Ventures Lab, a startup incubator and accelerator at Lehigh University. From 2011-2019, Dr. Hill was a member of the faculty at the U.S. Army War College. In 2017, he was appointed as the inaugural U.S. Army War College Foundation Chair of Strategic Leadership. Dr. Hill is also the founder and former Director of the Carlisle Scholars Program, and the founder and former Editor-in-Chief of WAR ROOM.
Stephen Gerras is Professor of Behavioral Science at the U.S. Army War College. Colonel (Retired) Gerras served in the Army for over 25 years, including commanding a light infantry company and a transportation battalion, teaching leadership at West Point, and serving as the Chief of Operations and Agreements for the Office of Defense Cooperation in Ankara, Turkey during Operations Enduring and Iraqi Freedom. He holds a B.S. from the U.S. Military Academy and an M.S. and Ph.D. in Industrial and Organizational Psychology from Penn State University.
The views expressed in this article are those of the author and do not necessarily reflect those of the U.S. Army War College, the U.S. Army, or the Department of Defense.
Photo Credit: Gemini image generator
I hope that these comments are relevant. Moravec’s Paradox suggests that computers have a hard time at target recognition and discrimination. In the current war between Russia and Ukraine, we see extensive jamming of systems and weapons. What that might mean is we tell a weapon to hit a target and it misses. Will the AI system know that?
We have a dilemma where we have to consider what is our OODA Loop’s speed compared to an enemy? This will put pressure on the decision makers.
We don’t see any mention of the fact that the human decision maker may not have the right training to make the calls. One site is Less Wrong which describes how to analyze problems.
We have to understand how our AI was trained. It may face an “Out of Sample Event” where it can’t make an informed decision because it lacks the training data.
We do need to understand whether our AI is secure from “Data Poisoning” making it useless or even harmful.
In my opinion, these are all topics to explore.
What Computers Can’t Do by Eli Zaretsky
Science ABC
Home » Technology
Moravec’s Paradox states that it is easy to train computers to do things that humans find hard, like mathematics and logic, but it is hard to train them to do things humans find easy, like walking and image recognition.
LESSWRONG
https://www.lesswrong.com/
OODA Loop
https://www.oodaloop.com/
Subverting AI’s Intellect: How to Thwart Data Poisoning: Finger pointing at digital wave Author: Prithiv Roshan Sudhakar Date Published: 1 January 2024
https://www.isaca.org/resources/isaca-journal/issues/2024/volume-1/subverting-ais-intellect-how-to-thwart-data-poisoning
The Future of Warfare Is Electronic: An audacious Ukrainian incursion into Russia shows why. Is the Pentagon paying enough attention? By Porter Smith and Nathan Mintz
https://www.wsj.com/opinion/the-future-of-warfare-is-electronic-ukraine-invasion-russia-is-the-pentagon-watching-73079a68
Re: the AI w/o humans thought, a question:
Will AI allow/be used to achieve “plausible deniability?” (“Sorry, the machine did it.” “Sorry, we now live in the world where it is the machines who make these decisions, not us, so you cannot blame us for either [a] these such current times and/or [b] the decisions that these machines — without human input — make in these such current times.)